- Статьи
- Internet and technology
- AI with a spark: neural network predicts forest fires with 87% accuracy%
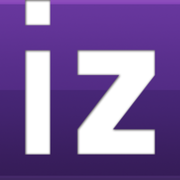
AI with a spark: neural network predicts forest fires with 87% accuracy%
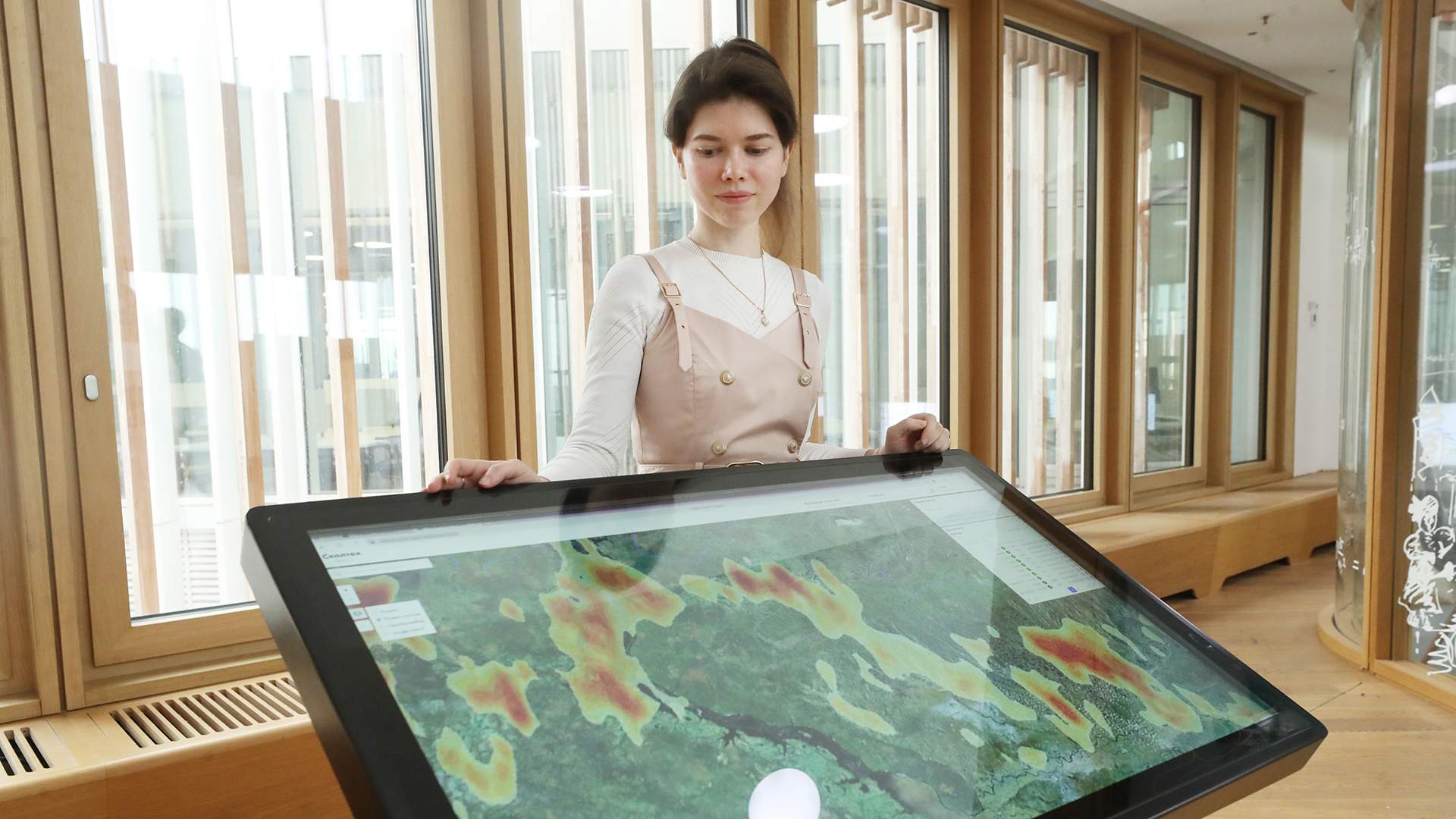
Russian scientists have trained neural networks to predict forest fires with an accuracy of 87%. Unlike its analogues, the system takes into account a variety of information, from weather data to population activity in the region, which improves the quality of predictions, the developers told Izvestia. Each region of the Russian Federation has its own unique features, so AI needs to be trained separately to work with different regions of the country. The system is currently being tested on Sakhalin. According to representatives of the Federal Forestry Agency, the department got acquainted with the development and asked the authors to finalize it. According to experts, the use of neural networks should improve the accuracy of forecasting wildfires, but in order to achieve qualitative improvements, a lot of work is needed.
AI for forest fire prediction
Skoltech specialists have developed an artificial intelligence-based system that predicts the occurrence of forest fires in Russian regions. Most similar developments make similar predictions based on the same type of data. In contrast, the new program takes into account heterogeneous information. Due to this, the accuracy of its forecasts can reach 87%. It depends on the quality of the information that the machine receives. The development is currently being tested in a number of regions, for example, on Sakhalin.
— We used machine learning technology. It was conducted on the basis of archived data for 10 years on whether or not there was a fire in a particular place at a particular time. The algorithm analyzed them and identified patterns by itself, according to which it is already possible to make predictions. Since each region of the country has its own characteristics, it is necessary to train AI for it separately. To begin with, we have selected several pilot regions. It took about one week to train the neural network for each of them," Svetlana Illarionova, head of the research group at the Skoltech Artificial Intelligence Center, told Izvestia.
The forecast is made five days in advance, as it is important for him to have reliable weather data. The developers have achieved prediction accuracy from 70% to 87%, depending on the region. This is sufficient for the practical use of the system so that responsible authorities can take measures to prevent fires. For example, to moisten the forest or close access to it for outsiders so as not to provoke a fire. In addition to regional authorities, the system can be useful for research institutes and other organizations whose activities may be affected by forest fires. Access to AI is available upon request. The system runs on Skoltech's internal computing clusters.
— When analyzing the situation, the neural network uses data on the Earth's surface, which is obtained using remote satellite observation methods. This is, for example, the type of vegetation and other surfaces. It is also taken into account in the vegetation index of vegetation cover, which reflects the condition of plants and their predisposition to a natural fire. It is calculated using a special mathematical model that converts spectral values from images to the target value," Svetlana Illarionova said.
The altitude above sea level, the direction of the slope, the slope, the population density and the distance from the roads are also taken into account. Terrain characteristics have an impact on the risk of fire. For example, steep mountain slopes are less prone to fire. Additional information about the distance from infrastructure facilities characterizes a person's ability to provoke an emergency as a result of careless handling of fire during a fire-prone period. All measurements are pre-placed in a common database, from where they are collected and further processed.
Information about total and potential evaporation, wind, air temperature, dew point temperature, and precipitation is taken from the weather forecast. These data allow us to assess the achievability of thresholds for ignition of various types of vegetation. The wind speed makes it possible to assess the predisposition of the environment to the spread of fire, that is, the likelihood that the resulting spark will not go out, but will grow into a strong flame. In addition, the AI calculates fire hazard indices, such as, for example, the Nesterov index, which reflects the balance of drying and moisturizing factors in the territory.
During the pilot implementation, which is being carried out in the Sakhalin Region, specialists helped adapt algorithms to local peculiarities and work out the necessary data analysis scenarios, Dmitry Evdokimov, director of the Digital Projects Department at the Ministry of Digital and Technological Development of the Sakhalin Region, told Izvestia.
— So far, we have tested the system mainly in winter, when the risk of fires is low, but even in these conditions, the platform already effectively collects and processes large amounts of satellite data, which makes it easier to predict natural threats. By the coming season, we plan to use all the functionality: both fire prevention and monitoring of other risks," he said.
Together with the developers, Sakhalin specialists will be able to fine—tune the AI modules even more precisely and achieve real benefits for the region in terms of timely response measures, ecosystem analysis and sustainable development of the territory, Dmitry Evdokimov added.
Development of fire hazard forecasting systems
— We are aware of the ongoing work of Skoltech to assess the likelihood of forest fires. Together with the Federal Aviation Security Service and the subordinate institute of VNIILM, we got acquainted with this model. Following the meeting, we noted that technical improvements are required. The new model does not take into account such important functions as mapping forest fires, monitoring the reliability of information about fire danger and the ability to generate reports," said Evgeny Pisarevsky, head of the Department of Forest Fire Protection at the Federal Forestry Agency.
Skoltech's work shows the direction in which fire hazard forecasting systems should be developed. So far, it looks more like a data manipulation technique. Fire hazard forecasts for various periods are regularly published and used by fire brigades. But their lack of reliability is not very high, said Konstantin Kobyakov, head of flora conservation projects at the Nature and People Foundation.
— Now the Nesterov index is most often used to assess fire danger, which shows how high the fire danger is in a particular place. Neural network technologies allow us to make forecasts more accurately than conventional indexes. Skoltech's work takes into account a fairly large amount of additional data that has not been available for a long time. Good coverage with satellite images appeared just recently and opened up new opportunities that developers used," he said.
Data on fire forecasting and assessment of the current level of fire danger should form the basis of management decisions. Based on them, it is possible to introduce or remove a special fire protection regime, increase the frequency of patrols, and impose time restrictions on visits to some of the most fire-prone places. Machine learning and the ability to work with a wide variety of open data expand these opportunities, says Grigory Kuksin, founder of the Center for Landscape Fire Prevention.
— But such models don't work well yet. And in this case, we cannot be sure of the high quality of forecasts. Part of the problem is that modern approaches and methods are applied to the topic without taking into account its specifics. For example, the authors apply the same approaches to determining fires by thermal anomalies (combining thermal points on different days into one fire or several different ones) in completely different natural areas. Such simplifications immediately greatly reduce the quality of the results obtained," he noted.
The same development team has created a similar system for the needs of the Ministry of Emergency Situations. It takes into account data from closed sources, so only employees of the department have access to it. Izvestia asked the Ministry about the quality of forecasting of this development.
Переведено сервисом «Яндекс Переводчик»